Artificial intelligence research methodology encompasses the approaches used to design, implement, evaluate, and deploy AI systems. It's a broad area with various approaches depending on the specific research goals. Here's a breakdown of key aspects:
Main research areas:
Theoretical research: Explores the fundamental principles and theoretical foundations of AI. This includes areas like knowledge representation, reasoning, learning, planning, decision-making, natural language processing, and computer vision.
Applied research: Focuses on developing practical solutions to real-world problems using established theoretical knowledge. This involves applications in various domains like healthcare, finance, manufacturing, transportation, and military.
Experimental research: Evaluates the performance and efficiency of AI systems. This includes data collection, preprocessing, model evaluation, and performance comparison techniques.
Research methodology stages:
Problem selection: Identifying a specific AI problem to address, considering its significance, feasibility, and potential impact.
Literature review: Examining existing research on the chosen problem to understand current state-of-the-art and inform your research direction.
Research plan formulation: Defining research objectives, methodology, timeline, budget, and potential challenges.
Research execution: Implementing the research plan, which may involve data gathering, model development, training, evaluation, and analysis.
Result presentation: Communicating research findings through publications, conferences, or presentations to share knowledge and contribute to the field.
Key elements of AI research methodology:
Problem selection: Choosing a problem with clear objectives, relevant to existing research, and potentially impactful.
Rigorous methodology: Employing appropriate research methods aligned with the problem and research goals, ensuring data quality, valid analysis, and reproducibility.
Evaluation and validation: Thoroughly evaluating the performance and limitations of your AI system using established metrics and benchmarks.
Ethical considerations: Addressing ethical concerns related to data privacy, fairness, bias, and potential societal impacts of AI.
Continuous evolution: AI research methodology is constantly evolving as new technologies and techniques emerge. Ongoing research in this area focuses on developing efficient and effective methods for tackling increasingly complex AI challenges.
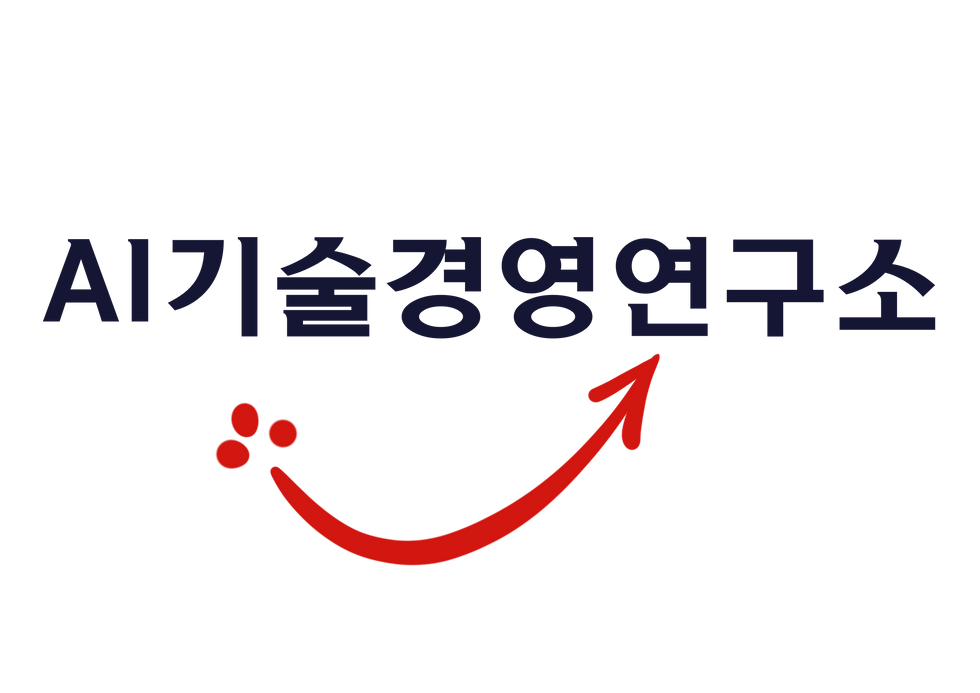
Comments